The Power of Data Annotation Tools and Platforms
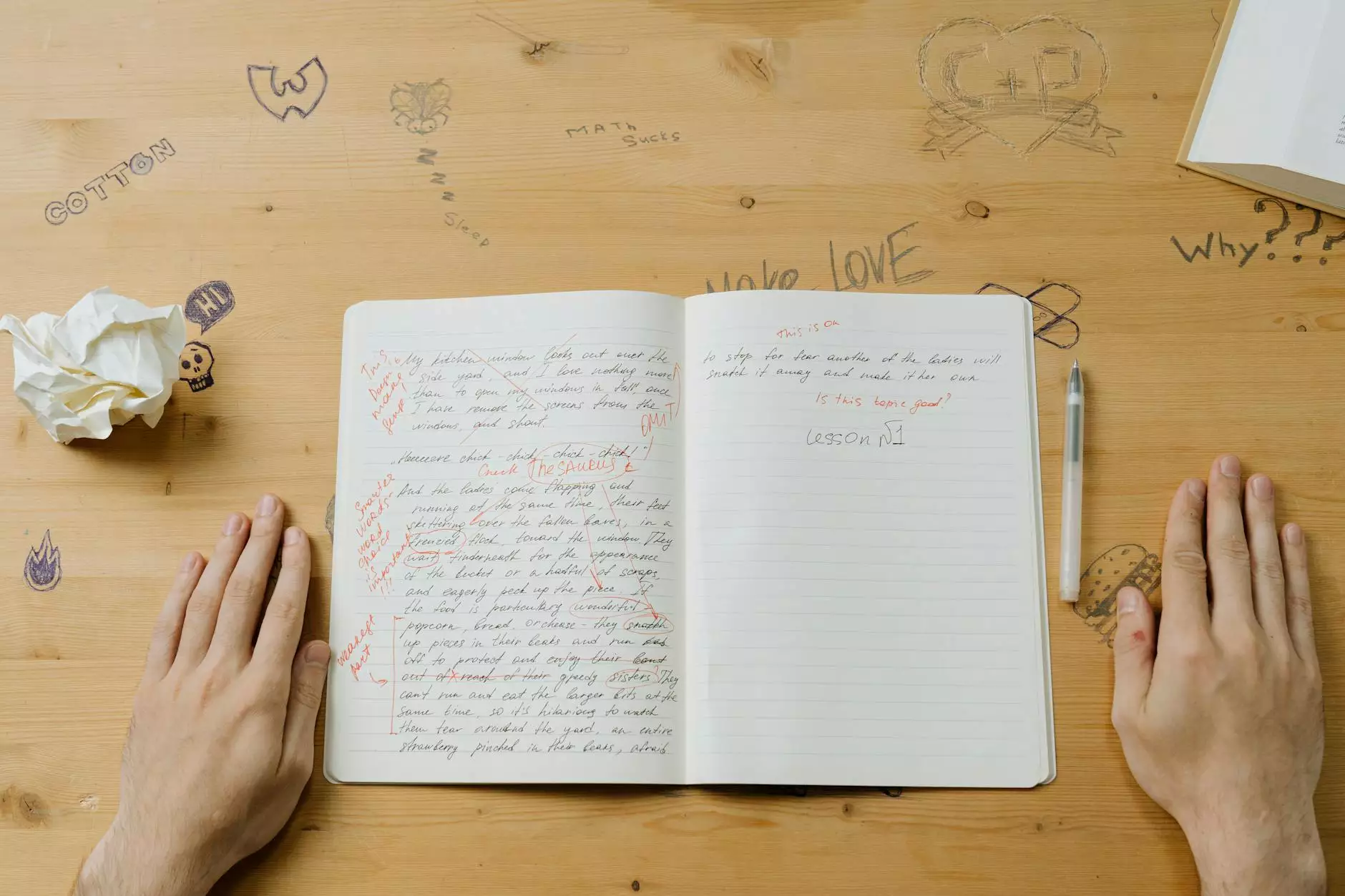
In the rapidly evolving landscape of technology and artificial intelligence, the significance of data annotation tools cannot be overstated. As businesses strive to leverage data for actionable insights, the need for accurate and efficient data labeling has emerged as a cornerstone of successful AI initiatives.
Understanding Data Annotation
Data annotation is the process of labeling data for machine learning and artificial intelligence models. This critical step involves highlighting important features in datasets, allowing algorithms to learn and make predictions based on structured information. Without proper data annotation, AI systems can falter, leading to less accurate outputs and poor decision-making.
The Business Impact of Data Annotation
Implementing robust data annotation strategies confers numerous benefits. Here are some key ways in which they impact your business:
- Enhanced Accuracy: Accurate data labeling leads to better model performance, resulting in more reliable AI applications.
- Increased Efficiency: Automated data annotation tools drastically reduce the time required to label datasets compared to manual methods.
- Scalability: Businesses can easily scale their data operations with a robust data annotation platform, handling larger datasets without compromising quality.
- Cost-Effectiveness: By minimizing the resources needed for data labeling, companies can lower operational costs while improving output quality.
Types of Data Annotation Tools
Data annotation tools come in various forms, each fulfilling different business needs:
1. Manual Annotation Tools
These tools allow human annotators to label data by hand. While they can provide high accuracy, they often require significant time and resources.
2. Automated Annotation Tools
These solutions use machine learning algorithms to automate the data labeling process. They are ideal for businesses that handle large volumes of data, especially when time is a critical factor.
3. Hybrid Annotation Tools
Combining the strengths of manual and automated tools, hybrid solutions facilitate human-in-the-loop workflows. This model enhances accuracy while enabling faster turnaround times.
Choosing the Right Data Annotation Platform
Selecting the right data annotation platform is crucial for your business’s success. Consider the following factors when making a decision:
- Ease of Use: The platform should be user-friendly, allowing seamless integration into your existing workflows.
- Collaboration Features: Look for tools that enable multiple users to work together efficiently, especially in team-oriented environments.
- Customizability: The ability to tailor the platform to your specific requirements enhances productivity and data quality.
- Support and Resources: Reliable customer support and extensive documentation can significantly improve your experience with the platform.
- Security Measures: Given the sensitivity of data, ensure that the platform implements robust security protocols to protect your information.
Real-World Applications of Data Annotation
The applications of data annotation are vast and varied, impacting multiple industries:
1. Healthcare
In healthcare, accurately labeled data is essential for developing AI-driven diagnostic tools and personalized medicine solutions.
2. Autonomous Vehicles
In the automotive industry, data annotation enables the training of AI systems for object and lane detection, enhancing the safety and reliability of self-driving cars.
3. E-Commerce
Data annotation helps enhance customer experiences through personalized product recommendations and better search functionalities on e-commerce platforms.
How to Optimize Your Data Annotation Strategy
To maximize the effectiveness of your data annotation efforts, consider implementing the following strategies:
- Define Clear Goals: Establish specific objectives for your data annotation projects to steer your efforts in the right direction.
- Invest in Training: Equip your annotation teams with proper training to ensure they understand the importance of accuracy and consistency.
- Regularly Review Quality: Periodically assess the quality of annotated data and implement feedback mechanisms to enhance performance.
The Future of Data Annotation
The future of data annotation is set to witness significant advancements driven by technological innovations. Here are some anticipated trends:
- AI-Assisted Annotation: The integration of AI into annotation processes will streamline workflows, making them faster and more efficient.
- Enhanced Accuracy with Machine Learning: As ML models evolve, they will become more adept at accurately labeling data, reducing reliance on manual inputs.
- Ethical Considerations: The focus on ethical AI practices will grow, leading to more transparent and responsible data annotation processes.
Conclusion
In conclusion, the importance of data annotation tools and platforms in the contemporary data-driven landscape cannot be overlooked. As businesses aim to harness the full potential of their data, investing in effective data annotation solutions is imperative. By understanding the nuances of data annotation, selecting the right tools, and optimizing your strategy, your organization can gain a competitive edge, drive innovation, and significantly enhance operational efficiency.
https://keylabs.ai/