Unleashing the Power of Machine Learning Labeling Tools for Businesses
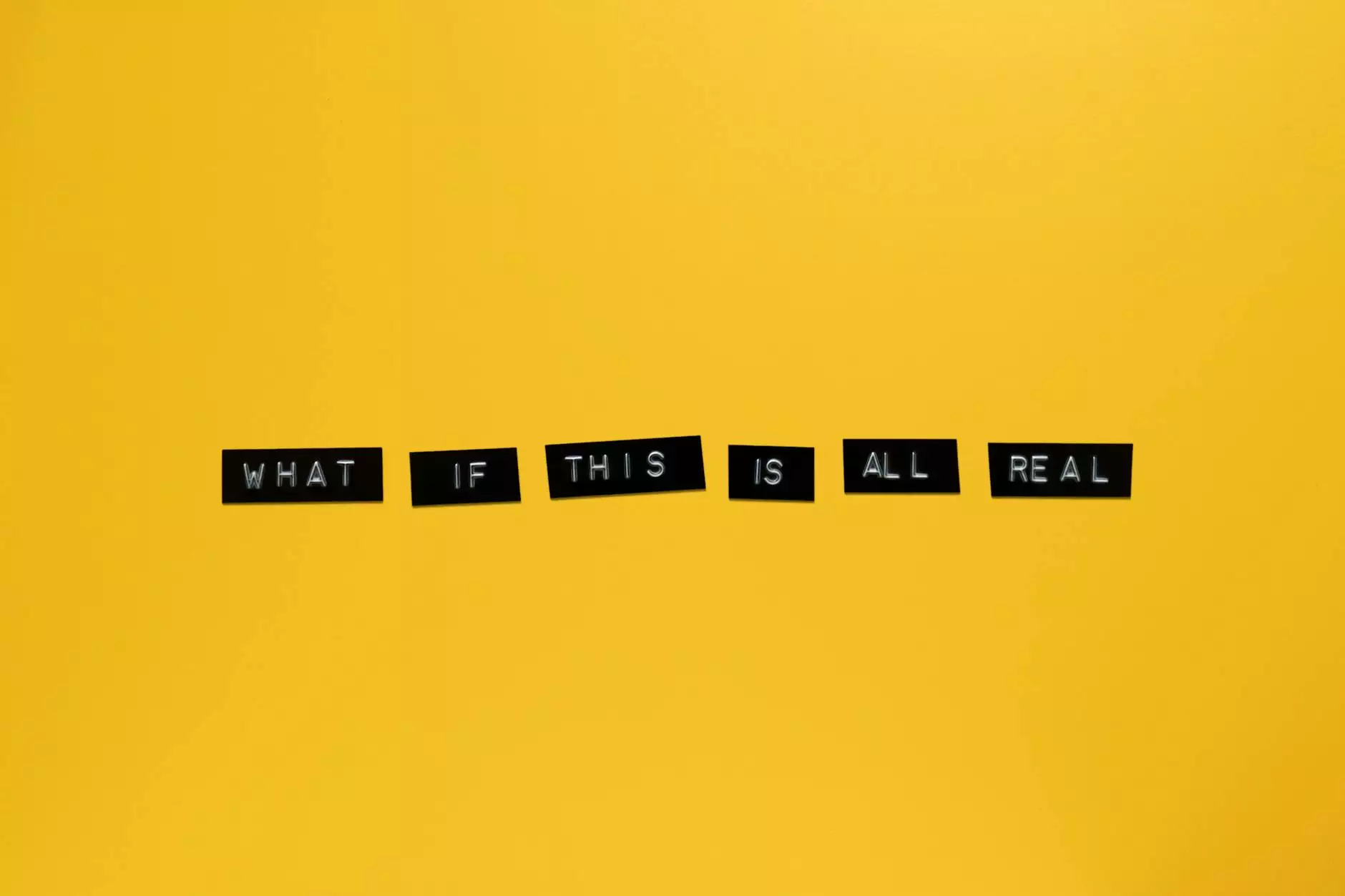
In today's fast-paced digital landscape, businesses are increasingly reliant on data to drive decisions, enhance customer engagement, and improve operational efficiency. The demand for high-quality, labeled data has never been greater, particularly in the realm of machine learning (ML). This is where the significance of employing efficient machine learning labeling tools comes into play.
What are Machine Learning Labeling Tools?
Machine learning labeling tools are specialized software solutions designed to assist in the data annotation process. They enable businesses to effectively label data, creating high-quality training sets necessary for building powerful machine learning models. The accuracy of predictions and the overall performance of ML algorithms largely depend on the quality of labeled data, making these tools essential for data-driven businesses.
Why Data Annotation is Critical for Businesses
Data annotation is the systematic labeling of data, which can include images, text, audio, and video. This process is critical for several reasons:
- Training Machine Learning Models: Labeled data is the backbone of all machine learning algorithms. Without it, even the most sophisticated ML models would fail to learn and generalize effectively.
- Enhancing Accuracy: Accurate data annotation directly impacts the performance of machine learning models, thereby improving their predictive capabilities.
- Streamlining Processes: Efficient data labeling reduces the time and cost associated with data preparation, allowing businesses to redirect resources to core activities.
- Facilitating Compliance: Businesses operate under various regulatory frameworks that require data transparency. Proper labeling ensures compliance and builds trust with stakeholders.
Benefits of Using Machine Learning Labeling Tools
Adopting machine learning labeling tools brings numerous advantages to organizations aiming to enhance their data annotation processes:
1. Improved Efficiency and Speed
One of the most significant benefits of using these tools is the remarkable improvement in efficiency and speed of data annotation tasks. Automation reduces manual labor, accelerating the overall data preparation process.
2. Enhanced Quality Control
Many advanced labeling tools integrate quality control mechanisms that ensure the highest standard of data accuracy. This is vital for creating dependable training sets that lead to successful ML outcomes.
3. Accessibility and Collaboration
Modern labeling tools often come with cloud-based functionalities, making data more accessible for teams across different locations. This fosters collaboration and facilitates real-time feedback, ultimately leading to better data quality.
4. Scalability
As businesses grow, so does their data. Machine learning labeling tools are designed to scale with the business, allowing for handling large volumes of data without sacrificing quality or efficiency.
Choosing the Right Machine Learning Labeling Tool
Selecting the right tool for your business can be daunting given the wide array of options available. Here are some critical factors to consider:
- Usability: The tool should have an intuitive interface, enabling users to navigate with ease.
- Integration Capabilities: Ensure the tool integrates seamlessly with your existing data systems and workflows.
- Feature Set: Look for features that cater to your specific data types, such as text, image, or audio annotation capabilities.
- Customer Support: Robust customer support is vital for troubleshooting and maximizing the potential of the tool.
- Cost: Evaluate the pricing structure to ensure it fits your budget while still meeting your labeling needs.
Types of Data Annotation in Machine Learning
Data annotation can take various forms depending on the type of data. Here are some common methods:
1. Image Annotation
This involves labeling images for object detection, image segmentation, and image classification tasks. Examples include drawing bounding boxes around cars in self-driving car datasets or segmenting pixels to identify different elements in a satellite image.
2. Text Annotation
It includes tasks such as named entity recognition, sentiment analysis, and text classification. Properly labeled text data is vital for building chatbots, recommendation systems, and other NLP applications.
3. Audio Annotation
Involves labeling audio clips for speech recognition and classification purposes. This is essential for developing voice-activated assistants and transcription services.
4. Video Annotation
This form of annotation encompasses labeling activities, events, or objects within video clips. It's frequently used in surveillance systems and activity recognition applications.
Integrating Machine Learning Labeling Tools into Your Workflow
To maximize the benefits of machine learning labeling tools, businesses need to integrate them effectively into their existing workflows. Here are a few strategies:
- Define Clear Objectives: Establish clear goals for data labeling projects to streamline processes and focus efforts.
- Train Your Team: Ensure that your team is well-trained in using the tools effectively to enhance productivity.
- Iterative Feedback: Implement a feedback loop to continuously improve labeling accuracy and efficiency.
- Monitor Performance: Regularly assess the performance of the labeling processes and the quality of the labeled data.
Case Studies: Success Stories with Machine Learning Labeling Tools
1. KeyLabs.ai: Transforming Data Annotation
KeyLabs.ai is revolutionizing the data annotation landscape with their innovative Data Annotation Tool. By leveraging advanced algorithms and user-friendly interfaces, they empower businesses to create high-quality labeled datasets promptly. Their success stories encompass a variety of industries, proving that effective data labeling can yield substantial operational benefits.
2. E-commerce Personalization
An e-commerce giant utilized machine learning labeling tools to enhance product recommendations. By accurately labeling customer interactions, they improved their recommendation algorithms, resulting in a 25% increase in sales through personalized marketing efforts.
3. Autonomous Vehicles
A leading automotive manufacturer employed machine learning labeling tools for annotating vast amounts of driving data. This led to the development of more reliable self-driving systems and a significant reduction in accidents during testing phases.
Future Trends in Machine Learning Labeling Tools
The future of machine learning labeling tools is promising. As AI and ML continue to evolve, we can expect to see:
- Increased Automation: Future tools will likely incorporate more sophisticated automation capabilities, minimizing human intervention in labeling tasks.
- Enhanced AI-Powered Suggestions: ML algorithms will help suggest labels based on patterns in data, further facilitating the annotation process.
- Mobile and Edge Computing: Expect a rise in mobile labeling solutions that allow for annotation on-the-go, enhancing flexibility.
- Real-Time Collaboration: Tools will enhance collaborative features, enabling teams to work more efficiently and effectively irrespective of location.
Conclusion
In conclusion, the use of machine learning labeling tools is paramount for businesses looking to harness the power of data. By ensuring accurate and efficient data annotation, companies can significantly enhance their machine learning models and, consequently, their business outcomes. As the demand for high-quality labeled data grows, investing in the right tools will position businesses for success in the competitive landscape. Embrace the future of data annotation with KeyLabs.ai’s innovative solutions and transform your data into actionable insights.